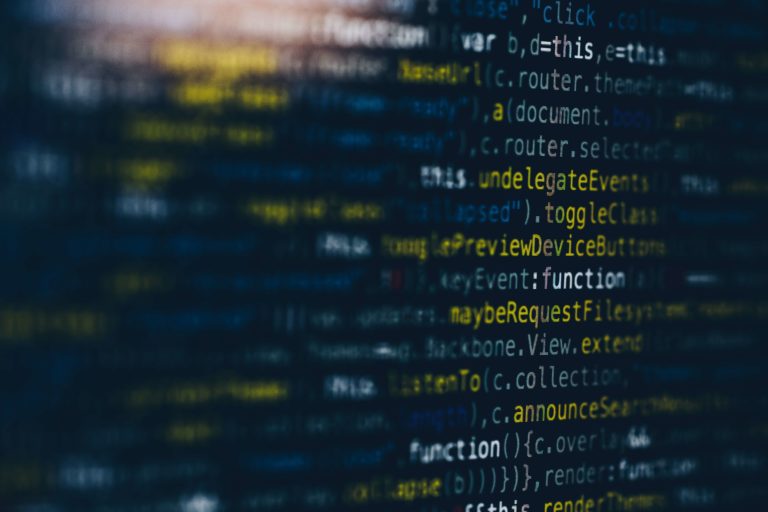
Bryan Smith is the Co-Founder and CEO of ThinkData Works, a Toronto-based technology company whose platform is used by large global organizations to process and refine data into usable products. They host one of the world’s largest repositories of publicly available data and enable their clients to significantly increase the amount of information they use to run their business.
Prior to founding ThinkData Works, Bryan worked for the Canadian Government as a Sr. Policy Advisor to the President of the Treasury Board of Canada where he helped implement the Government’s “Value for Money Ethic”, leading to over $7B in annual savings and the first transition from deficit to surplus in the past 20 years.
One of the many ways data can be used in financial services is to combat organized crime by tracking and monitoring trends in global trade. ThinkData Works recently partnered with Dun & Bradstreet to help financial institutions improve their anti-money laundering (AML) programs with a data enrichment solution. Major banks including Scotiabank are already leveraging ThinkData’s data solution for their AML initiatives.
In this Q+A, we chat with Bryan about how data can be harnessed to support AML efforts at Canadian banks.
What is ThinkData Works?
BS: ThinkData Works builds technology to help organizations get more value out of data. Our Data Catalog Platform lets organizations index and manage all their data from a central location without needing to redesign their data ecosystem. With tooling designed for both data and business professionals, the ThinkData Catalog Platform is a user-friendly API-based mission control system where teams can find, share, and collaborate on the data they need to power business decisions.
Since ThinkData Works was founded in 2014, the company has grown to work with some of Canada’s biggest banks, including RBC and Scotiabank. As a data software company, how do you provide value to financial institutions?
BS: Our team works with financial institutions to help streamline their data-driven processes. Financial institutions are some of the most data-smart organizations in the world – as quick adopters of big data and analytics, they support large teams of data scientists, engineers, and business analysts who use data to support innovation initiatives. Our platform gives their data teams a standard place to find and monitor the health of their datasets, then effortlessly integrate the data into their preferred data science toolkits or business intelligence applications.
The United Nations Office on Drugs and Crime (UNODC) estimates that between 2 and 5% of global GDP is laundered each year. In terms of anti-money laundering (AML), what is the responsibility for banks in minimizing this number?
BS: While banks have a responsibility to implement AML initiatives, regulatory bodies often evaluate them by how much money they spend on these initiatives, not whether they’re effective. Traditionally, banks use the internal data they collect from their account holders to spot irregular, concerning, or illegal activity. The problem is often the information they have only shows a part of a much larger picture; it doesn’t do enough to mitigate the impact of more sophisticated criminals, who launder money through international networks and asset purchases which are more complex and require more datasets to track accurately. To stem the tide of money laundering activity, banks should be looking to evolve the process they’ve been using.
How does ThinkData Works support banks in their AML efforts?
BS: ThinkData supports banks by providing new external sources of data that the bank can use alongside their internal data to create an AML database that looks beyond the traditional data points they have, and gives the bank a more holistic view of their customer behaviour. External data like global trade data, new commercial licenses, real estate purchases, and leaked offshore investment information can be used alongside a bank’s internal data to generate a database that can then be queried and forensically scrutinized to spot more suspicious activity or raise the confidence on potential money laundering activity, leading to better processes and more streamlined AML initiatives.
As banks adopt AI and automation into their AML systems, is there still a need for a human element? If so, what is the human role?
BS: As more data flows into a bank and intelligent models start to develop, the human element will become more, not less, important. AI efforts need to be rigorously tested for bias – as the data powering early AI initiatives will be sourced from the public domain, it’s imperative for banks to maintain a human line of sight into what the data is, how it’s being used, and when machine learning might generate bias in the algorithm.
The common misconception about AI is that it will provide answers – it won’t, and it shouldn’t. AI is successful if it enables humans to forensically look at the output generated from a model and make sound, ethical judgements based on their own experience and knowledge base outside of the data. The goal for banks should be to evolve their data ecosystem to support the consumption and management of new data – and provide analysts with more tools, better data, and a sandbox environment where they can test models and see what kind of results come back.
Beyond AML and other financial crimes, how can data help organizations achieve their ESG objectives?
BS: Right now there is a big focus on governance, especially for organizations who have a data mandate in place. The problem they’re facing is the explosion of their own data, combined with the overwhelming availability of new external sources of data. To manage the overwhelming amount of data and remain compliant to evolving regulation, organizations need to understand what data they have, where it’s coming from, and what it’s powering.
In terms of environmental objectives, by effectively indexing and managing data, organizations will also be able to determine which data sources are more environmentally friendly and sustainable, letting them reconfigure their internal supply chain to better focus on ESG initiatives without compromising on quality or internal impact.
Looking towards the future of big data in financial services, what are emerging trends/technology to watch?
BS: A lot of people are currently talking about data mesh and data fabric as two trends that will redefine the state of affairs for financial services. My opinion is that these trends are the latest in a long line of “catch-all” solutions that aim to solve all the problems you have with data in one piece of technology. Innovative technology like AI and machine learning are the result of a well executed data strategy, not the start of one, and any solution, framework, or trend that promises to automatically extract value from a data ecosystem that hasn’t been properly set up is essentially promising alchemy. There’s no problem with data mesh or data fabric as ideas, but they should be considered after financial organizations develop battle-tested data systems that are capable of cataloging and managing all their data, bringing in new forms of data, and providing an equal playing field for everyone who needs to use the data to generate value.
Leave a Reply